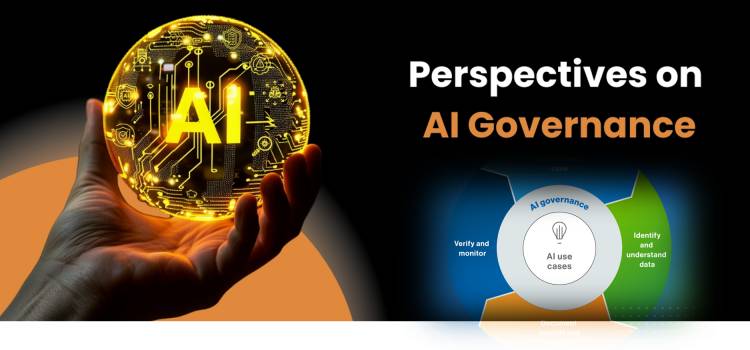
AI Governance Framework: Ensuring Responsible AI development and deployment
AI Governance Framework: ensuring responsible AI development and deployment
Introduction
Artificial intelligence (AI) is transforming industries and societies, but its rapid development brings challenges related to ethics, safety, bias, and accountability. An AI governance framework sets out policies, regulations, and guidelines to ensure AI systems are transparent, fair, and aligned with human values.
Table of Contents
AI Governance Framework: ensuring responsible AI development and deployment. 1
1. Key principles of AI governance. 1
1.1. Fairness and bias reduction. 1
1.2. Transparency and clarity. 1
1.3. Privacy and data protection. 1
1.4. Responsibility and accountability. 1
2. Components of an AI governance framework. 2
2.1. AI policy and regulation. 2
2.2. AI Ethics and Compliance Committees. 2
2.3. Risk management and impact assessment 2
2.4. Human-involved systems (HITL) 2
2.5. AI monitoring and auditing. 2
2.6. Responsible AI deployment. 2
3. Global AI governance initiatives. 2
European Union Artificial Intelligence Law.. 2
3.2. UNESCO Recommendation on AI Ethics. 3
3.4. AI regulations in China. 3
3.5. AI Governance Strategy in India. 3
4. Challenges in AI governance. 3
5. The future of AI governance. 3
Below are 10 key points that are typically covered: 4
________________________________________________
1. Key principles of AI governance
An AI governance framework should be built on core principles that guide the development and use of ethical AI:
1.1. Fairness and bias reduction
- AI should be free from discrimination and bias.
- Regular audits and fair testing are needed to prevent biased decision-making.
1.2. Transparency and clarity
- AI models should be interpretable, so users can understand how decisions are made.
- Organizations should demonstrate the use of AI, especially in key areas such as finance and healthcare.
1.3. Privacy and data protection
- AI must comply with data protection laws such as GDPR (Europe) and CCPA (California).
- User data must be anonymized, encrypted, and stored securely.
1.4. Responsibility and accountability
- Organizations using AI must be held accountable for its consequences.
- There should be clear procedures to address AI errors and vulnerabilities.
1.5. Safety and security
- AI systems must be tested for vulnerabilities and protected from cyber threats.
- AI must be aligned with human safety standards to avoid unintended consequences.
________________________________________________
2. Components of an AI governance framework
A well-structured AI governance framework consists of multiple layers:
2.1. AI policy and regulation
- Governments and international organizations (e.g., OECD, UNESCO, and the EU AI Act) set AI governance policies.
- Laws regulate AI applications in finance, healthcare, criminal justice, and autonomous systems.
2.2. AI Ethics and Compliance Committees
- Companies should have internal AI ethics boards to review AI development.
- External audits ensure compliance with AI ethical guidelines.
2.3. Risk management and impact assessment
- AI risk assessment should be conducted before deployment.
- High-risk AI applications (e.g., facial recognition, automated hiring) require strict oversight.
2.4. Human-involved systems (HITL)
- Critical AI decisions (e.g., loan approval, medical diagnosis) should include human oversight.
- Hybrid AI-human models ensure that AI complements human judgment rather than replacing it.
2.5. AI monitoring and auditing
- AI models should undergo continuous monitoring to detect unintentional bias and security vulnerabilities.
- AI auditing tools assess compliance with ethical and legal standards.
2.6. Responsible AI deployment.
- Organizations should ensure that AI aligns with corporate values and social impact goals.
- Developers should follow frameworks such as the NIST AI Risk Management Framework for responsible AI deployment.
________________________________________________
3. Global AI governance initiatives
European Union Artificial Intelligence Law
- Classifies AI applications by risk (unacceptable, high, limited, minimal).
- Bans harmful AI practices such as social scoring and biometric mass surveillance.
3.1. OECD AI Principles
- Calls for AI to be inclusive, sustainable, and human-centred.
- Encourages transparency and accountability in AI development.
3.2. UNESCO Recommendation on AI Ethics
- Advocates for AI governance based on human rights and ethical considerations.
3.3. USAI Bill of Rights
- Focuses on AI safety, data privacy, preventing algorithmic bias, and user rights.
3.4. AI regulations in China
- AI-generated content requires labeling and compliance with censorship laws.
- Focuses on government control over AI implementation.
3.5. AI Governance Strategy in India
- Prioritizes AI for social good, ensuring responsible adoption of AI in healthcare and education.
________________________________________________
4. Challenges in AI governance
Despite global efforts, AI governance faces several challenges:
- Lack of standardized regulations: There are inconsistent AI policies across different countries.
- Bias and ethical dilemmas: AI models still show discrimination in hiring, credit scoring, and policing.
- AI liability issues: It is unclear who is responsible when AI systems fail.
- Rapid advances in AI: Regulations struggle to keep up with the rapid development of AI.
- Security threats: AI-driven cyberthreats (deepfakes, automated hacking) require strong defenses.
________________________________________________
5. The future of AI governance
AI governance will evolve with new advances in AI research, and future frameworks will focus on:
- Strong international cooperation: A unified global AI governance framework could emerge.
- Explainable and trustworthy AI: AI models will be more interpretable for users.
- Strict AI liability laws: Companies will be held accountable for harm caused by AI.
- AI for the public good: Ethical AI policies will promote AI solutions to societal challenges.
_______________________________________________________________
10 main points:
An AI governance framework provides a structure for developing, deploying, and monitoring AI systems responsibly and ethically.
Below are 10 key points that are typically covered:
- Ethical principles: This sets out a set of core ethical principles to guide the development and use of AI. These often include fairness, transparency, accountability, confidentiality, and human oversight.
- Risk management: This describes the process of identifying, assessing, and mitigating potential risks associated with AI systems, such as bias, discrimination, and safety concerns.
- Data governance: This describes rules and regulations for collecting, storing, and using data responsibly and ethically, ensuring data quality, privacy, and security.
- Transparency and clarity: This emphasizes the importance of understanding how AI systems work and making their decision-making processes clear and explainable.
- Responsibility and accountability: Assigns clear roles and responsibilities for the development, deployment, and outcomes of AI systems, ensuring accountability for their actions.
- Human oversight and control: Establishes mechanisms for human oversight and control over AI systems, allowing for intervention and correction when necessary.
- Compliance and regulation: Addresses relevant laws, regulations, and industry standards related to AI, ensuring compliance and adherence to legal requirements.
- Monitoring and evaluation: Describes the process of monitoring the performance and impact of AI systems, assessing their effectiveness, and identifying areas for improvement.
- Collaboration and communication: Promotes collaboration and communication among stakeholders, including developers, users, and affected parties, to achieve a shared understanding and address concerns.
- Continuous improvement: Emphasizes the importance of continuous improvement and adaptation of the AI governance framework based on experience, feedback, and the development of best practices.