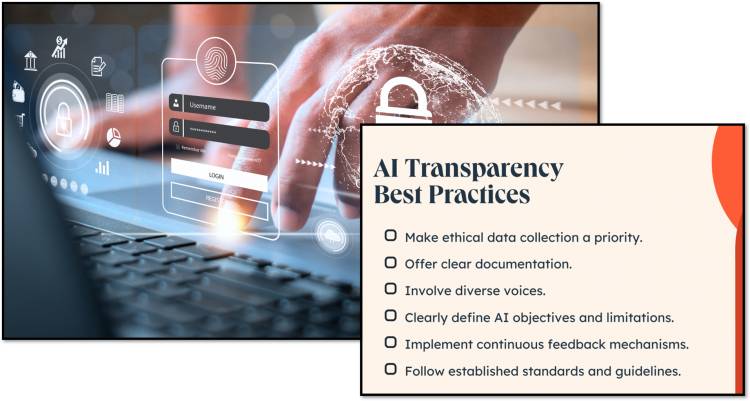
AI Transparency and Reporting
Detailed information in AI Transparency and Reporting
Transparency and reporting in AI are crucial to building trust, ensuring accountability, and promoting the development and deployment of responsible AI.
Below is a detailed description of this important topic:
Table of Contents
Detailed information in “AI Transparency and Reporting”. 1
Why is AI transparency important?. 1
How to achieve transparency in AI 1
Challenges to achieving transparency in AI 2
The Future of AI Transparency and Reporting. 2
10 key points about transparency and reporting in AI: 3
4. Facilitates understanding: 3
9. User-friendly interfaces: 4
Research paper on “Transparency and Reporting in AI”. 4
3. Transparency in data and algorithms: 4
4. Reporting and communication: 5
5. Moral and social implications: 5
Possible research directions. 5
What is AI transparency?
AI transparency refers to the openness and clarity of how AI systems operate, make decisions, and impact individuals and society. This includes providing information on the following aspects:
- Data: What data was used to train the AI model? How was it collected and processed? Are there biases or limitations in the data?
- Algorithm: How does the AI model work? What algorithms and techniques are used to process data and make decisions?
- Decision-making process: How does the AI system arrive at a particular outcome or decision? What factors are taken into account and how are they weighted?
- Limitations: What are the limitations of the AI system? What are its potential biases, errors, or unintended consequences?
- Purpose: What is the intended purpose of the AI system? How is it used, and who is responsible for its use?
Why is AI transparency important?
- Builds trust: When people understand how AI systems work, they are more likely to trust them. Transparency helps dispel fears and misconceptions about AI being a “black box.”
- Ensures accountability: Transparency allows AI systems and their developers to be held accountable for their actions and outcomes. If an AI system makes a mistake or causes harm, it is important to understand why and who is responsible.
- Promotes ethical AI: Transparency is essential to identifying and mitigating biases and ethical concerns in AI systems. By understanding how AI models are trained and how they make decisions, we can ensure that they are fair, unbiased, and aligned with human values.
- Facilitates understanding and acceptance: Transparency helps people understand the benefits and limitations of AI, making them more likely to accept and use AI systems responsibly.
- Supports regulation: Transparency is crucial to developing effective regulations and standards for AI. Regulators need to understand how AI systems work to ensure they are safe and beneficial.
How to achieve transparency in AI
- Explainable AI (XAI): Develop AI models that can provide human-understandable explanations for their decisions and actions.
- Interpretable AI: Focus on creating AI models that are inherently understandable, allowing humans to understand their inner workings.
- Documentation: Maintain clear and comprehensive documentation on the data, algorithms, and decision-making processes of AI systems.
- Auditing: Implement audit procedures for AI systems to ensure they are working as intended and are free from bias.
- Transparency reporting: Publish reports that provide information about the development, deployment, and impact of AI systems.
- User interface: Design user interfaces that provide clear and informative explanations about how to use AI.
- Education: Educate users and stakeholders about AI and how it works, empowering them to make informed decisions.
Challenges to achieving transparency in AI
- Complexity of AI models: Many AI models, especially deep learning models, are complex and difficult to understand.
- Trade-off between accuracy and interpretability: There is often a trade-off between the accuracy of an AI model and its interpretability. More complex models are more accurate but less interpretable.
- Protection of intellectual property: Companies may be reluctant to disclose details about their AI models for fear of trade secrets being exposed.
- Lack of standards: There is a lack of standard metrics and frameworks for measuring and reporting AI transparency.
Reporting on AI
Reporting on AI involves communicating information about AI systems to various stakeholders, including users, regulators, and the public. This may include:
- Technical details: Information about the data, algorithms, and architecture of AI models.
- Performance metrics: Data related to the accuracy, reliability, and performance of AI systems.
- Ethical considerations: Discussion of potential biases, risks, and ethical implications of AI systems.
- Impact assessment: Reporting on the social, economic, and environmental impacts of AI systems.
The Future of AI Transparency and Reporting
As AI becomes more mainstream, transparency and reporting will become even more important. We can expect to see:
- Increased regulation: Governments and regulatory bodies will likely introduce new regulations and standards for AI transparency and reporting.
- Advances in XAI: Researchers will continue to develop new techniques to make AI models more interpretable and interpretable.
- Industry standards: Industry organizations are likely to develop standards and best practices for AI transparency and reporting.
- Public awareness: The public will become more aware of the importance of AI transparency and demand greater accountability from AI developers and implementers.
By ranking transparency and reporting, we can confirm that AI is developed and used responsibly, morally, and for the benefit of all.
10 key points about transparency and reporting in AI:
- Figures trust: Transparency in AI promotes trust by showing how systems work, removing the perception of a “black box.”
- Confirms accountability: Clear reporting makes it possible to understand AI decisions, ensuring accountability in the event of error or harm.
- Promotes ethical AI: Transparency reveals potential biases in data or algorithms, which is important for the development of fair and ethical AI.
- Facilitates understanding: When people understand how AI works, they are more likely to accept it and use it responsibly.
- Supports regulation: Transparency is key for regulators to understand AI systems and create effective rules for their use.
- Understandable AI (XAI): Focus on developing AI that can explain its decisions in a way that humans can understand.
- Documentation is key: Maintain clear records of the data, algorithms, and processes involved in AI systems.
- Auditing and reporting: Implement methods to audit AI and publish reports on its development, use, and impact.
- User-friendly interfaces: Design interfaces that explain how AI is being used in a clear, accessible way.
- Ongoing development: Transparency and reporting in AI are emerging fields, requiring continuous improvement and adaptation.
Research paper on “Transparency and Reporting in AI”
You are looking for research on AI transparency and reporting! This is a hot topic, so there is a lot going on. Here is a breakdown of the key areas and some possible research directions:
Key research areas
1. Explainable AI (XAI):
- Developing XAI techniques: Researching new methods to make AI decisions understandable, such as attention mechanisms, rule extraction, or fact-based explanations.
- Evaluating XAI: Studying the effectiveness of different XAI techniques for different users and tasks, and how they affect trust and decision making.
- XAI for complex models: Addressing the challenge of describing highly complex AI models, such as deep neural networks.
2. Interpretable AI:
- Building interpretive models: Designing AI models that are inherently transparent, such as decision trees or rule-based systems.
- The trade-off between accuracy and interpretability: investigating the trade-off between accuracy and interpretability in AI models.
- Applications of interpretable AI: exploring how interpretable AI can be used in important areas such as healthcare or finance.
3. Transparency in data and algorithms:
- Data provenance: investigating ways to track and document the origin and processing of data used to train AI models.
- Algorithmic transparency: developing techniques to make AI algorithms more understandable, including visualising how they work or simplifying their logic.
- Bias detection: investigating how transparency can help identify and mitigate biases in data and algorithms.
4. Reporting and communication:
- Transparency framework: developing a standard framework for reporting on AI systems, including what information to disclose and how to present it.
- Effective communication: investigating how to communicate information about AI to different audiences, including technical experts, policy makers and the general public.
- Impact assessment: investigating methods to assess and report the social, economic and ethical impacts of AI systems.
5. Moral and social implications:
- Transparency and trust: studying the relationship between AI transparency and trust in AI systems.
- Responsibility and accountability: investigating how transparency can enable accountability for AI decisions and actions.
- Transparency and fairness: investigating how transparency can help ensure fairness and prevent discrimination in AI systems.
Possible research directions
- User-centered transparency: investigating how to tailor AI descriptions and reporting to the needs and understanding of different users.
- Transparency in practice: conducting case studies to examine how organizations are implementing AI transparency and reporting in real-world settings.
- Transparency and regulation: investigating the role of transparency in AI regulation and governance.
- Transparency and Public Discourse: Study how transparency can contribute to informed public discourse about AI and its implications.
- AI Transparency and Safety: Investigate how transparency can help identify and mitigate potential risks and harms associated with AI systems.
Where to Get Research?
- Academic Journals: Find articles in journals related to AI, computer science, ethics, law, and social sciences.
- Conferences: Explore conference proceedings related to AI, machine learning, and related fields.
- Research Institutions: Check out the websites of universities and research labs working on AI transparency and reporting.
- Industry Reports: See reports published by companies, NGOs, and government agencies on AI ethics and transparency.
- Online Resources: Explore websites and blogs dedicated to AI ethics, transparency, and responsible AI.
Remember to refine your search based on specific areas of interest within AI transparency and reporting. Good luck with your research!
_____________________________________________________________________