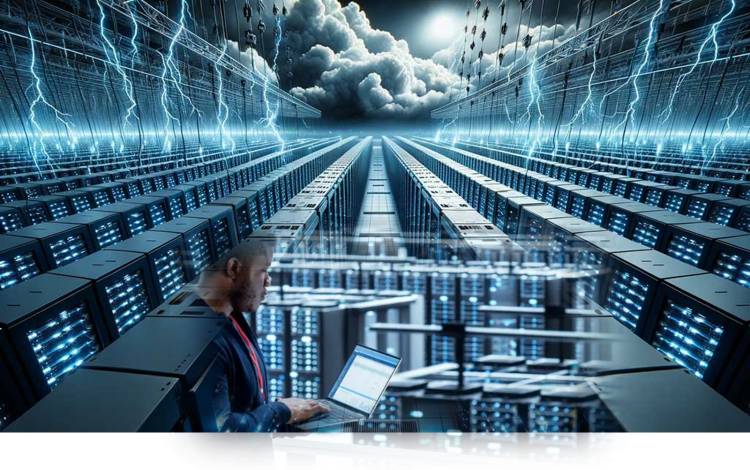
High energy consumption with AI
High energy consumption with AI in detailed information
AI, while offering immense development potential, involves significant energy consumption. This is mainly due to the complex calculations required to train and run AI models, especially those involved in machine learning and deep learning.
Below is a breakdown of the factors that contribute to the high energy consumption of AI:
Table of Contents
High energy consumption with AI in detailed information. 1
4. Data Storage and Transfer: 2
10 Key Points of AI’s “High Energy Consumption”. 3
1. Computationally intensive: 3
4. The thirst for generative AI: 3
5. Data Storage and Movement: 3
Here are five key questions and answers about AI’s high energy consumption: 4
Q1: Why is AI so energy intensive?. 4
Q2: What are the main contributors to AI’s energy impact?. 4
Q3: What are the environmental consequences of AI’s energy consumption?. 4
Q4: Are there ways to reduce AI energy consumption?. 5
Q5: What is the future of AI energy consumption and what can be done?. 5
1. Computational control:
- Training AI models: Training complex AI models, such as those used in natural language processing (e.g. ChatGPT) or image recognition, involves processing large data sets through billions of calculations. This process can take weeks or months and requires thousands of specialized computing chips (GPUs or TPUs) working concurrently in large data centers.
- Running AI models: Even after training, running these models for tasks such as generating text or analyzing images requires significant computational power, which contributes to ongoing energy usage.
2. Data Centers:
- Hardware and Cooling: Data centers, the physical infrastructure that enables AI, consume a lot of electricity not only to power the calculations but also to maintain the cooling systems required for the hardware to run efficiently around the clock.
- Data Center Growth: The rise of AI is rapidly increasing the number of data centers globally, leading to an increase in overall energy consumption.
3. Model Complexity:
- Size and Complexity: As AI models grow in size and complexity, so do their energy requirements. Larger models with more parameters require more computational power to both train and run them.
- Generative AI: Generative AI, which creates new content such as text, images, or code, is energy intensive due to the complexity of the algorithms involved.
4. Data Storage and Transfer:
- Storage: Storing the large data sets used in AI training requires robust storage solutions, which also consume electricity.
- Data movement: Transferring data between storage and processing units, known as data movement, is another high energy consumption point.
5. Environmental impact:
- Carbon footprint: AI’s high energy consumption results in a significant carbon footprint, which contributes to greenhouse gas emissions and climate change.
- Resource scarcity: AI also puts pressure on non-renewable resources such as zinc and mercury, which are mined to build the infrastructure needed to train and run these models.
- Examples of high energy consumption in AI:
- Training large language models: Training a large language model like GPT-3 can consume as much electricity as powering a small city for a day.
- Generative AI tasks: Creating an image using an AI image generator can consume as much energy as fully charging a smartphone.
- AI-powered search: Interacting with an AI-powered search engine like ChatGPT can use 10 times more energy than a standard Google search.
- Addressing the challenge of energy consumption:
- While the energy consumption of AI is a major concern, efforts are being made to reduce its
- impact:
- Efficient hardware: Developing more energy-efficient hardware, such as specialized AI chips and neuromorphic computing systems, can significantly reduce energy consumption.
- Model optimization: Techniques such as model compression and pruning can reduce the size and complexity of AI models, making them more energy-efficient.
- Renewable energy: Powering data centers with renewable energy sources, such as solar and wind, can help reduce the carbon footprint of AI.
- Sustainable AI practices: Implementing sustainable AI practices, such as optimizing algorithms for energy efficiency and reusing trained models, can further reduce energy consumption.
Conclusion:
The high energy consumption of AI is a complex problem involving multiple contributing factors. While the benefits of AI are undeniable, addressing the challenge of its energy consumption is crucial to ensuring its sustainable development. By investing in energy-efficient hardware, improving AI models, and adopting sustainable practices, we can harness the power of AI while reducing its environmental impact.
10 Key Points of AI’s “High Energy Consumption”
You got it! Here are 10 key points that summarize AI’s high energy consumption:
- Computationally intensive: Training and running AI models, especially those used in large language models and generative AI, requires a lot of computational power due to complex calculations.
- Data center dominance: AI relies heavily on data centers, which are themselves huge consumers of electricity to power the hardware and maintain the necessary cooling systems.
- Model complexity: As AI models grow in size and complexity, their energy demand increases rapidly. Larger models with more parameters require significantly more computational resources.
- The thirst for generative AI: Generative AI, which creates new content such as text, images, and code, is very energy intensive due to the complex nature of its algorithms.
- Data Storage and Movement: Storing large data sets used in AI training and transferring data between storage and processing units play a significant role in energy consumption.
- Environmental Impact: AI’s high energy consumption results in a substantial carbon footprint, contributing to greenhouse gas emissions and exacerbating climate change.
- Resource Scarcity: The infrastructure required for AI development and deployment relies on non-renewable resources such as zinc and mercury, further damaging the environment.
- Training vs. Inference: While training AI models is energy-intensive, the ongoing use (creation) of these models also requires significant computational power, increasing current energy consumption.
- Lack of Transparency: There is often a lack of transparency regarding the exact energy usage of specific AI models and processes, making it difficult to fully assess environmental impacts.
- Need for solutions: Addressing AI energy consumption requires a multi-pronged approach, including developing more energy-efficient hardware, improving AI models, and using renewable energy sources.
Q/A
5 key questions and answers about AI’s high energy consumption:
Q1: Why is AI so energy intensive?
AI, especially deep learning, relies on training large models on vast data sets. This involves complex calculations, which require powerful hardware and ample cooling in data centers. The sheer scale of data processing and the complexity of algorithms are the main drivers of high energy consumption.
Q2: What are the main contributors to AI’s energy impact?
There are several factors that contribute to this: the computational power required to train and run models, the energy requirements of data centers (including hardware and cooling), the size and complexity of AI models, the energy required for data storage and transfer, and the use of non-renewable energy sources to power these processes.
Q3: What are the environmental consequences of AI’s energy consumption?
High energy consumption results in a significant carbon footprint, which contributes to greenhouse gas emissions and climate change. It also leads to a shortage of non-renewable resources used in manufacturing hardware for AI systems.
Q4: Are there ways to reduce AI energy consumption?
Yes, several approaches are being explored: developing more energy-efficient hardware (such as specialized AI chips), optimizing AI models to reduce their size and complexity, powering data centers with renewable energy, and implementing more sustainable AI practices (such as reusing trained models).
Q5: What is the future of AI energy consumption and what can be done?
Without intervention, AI energy consumption is likely to increase significantly as models become larger and more popular. Addressing this problem requires a multi-pronged approach: continued research into energy-efficient hardware and algorithms, increased transparency about energy use, and a shift toward sustainable AI practices across the industry. Individual users can also contribute by being aware of the AI applications they use and supporting companies that prioritize energy efficiency.