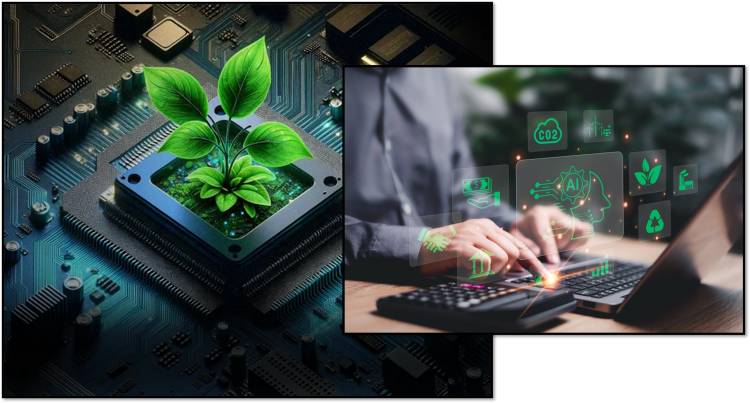
AI in Green Computing
Detailed Information on “AI in Green Computing”
Artificial Intelligence in Green Computing: A Detailed Overview
Green computing, also known as sustainable computing, focuses on the efficient design, manufacturing, and use of computing resources to reduce their environmental impact. AI (artificial intelligence) plays a crucial role in green computing by optimizing power consumption, reducing e-waste, and improving overall performance in data centers, hardware design, and software applications.
Table of Contents
Detailed Information on “AI in Green Computing”. 1
1. What is AI in Green Computing?. 1
2. Major applications of AI in green computing. 1
3. Benefits of AI in green computing. 2
4. Challenges of AI in green computing. 2
5. The future of AI in green computing. 2
Here are 10 key takeaways from “AI in Green Computing”: 3
1. Environmental impact of AI: 3
2. Green computing as a solution: Green. 3
4. Energy-efficient hardware: 3
5. Renewable energy for data centers: 3
7. Innovations in data center cooling: 3
8. Lifecycle and e-waste reduction: 3
9. AI in sustainable practices: 3
White Pages Research on “AI in Green Computing”. 3
1. Energy Efficiency in AI Systems: 4
2. AI for Sustainable Data Centers: 4
3. AI in resource management: 4
4. AI for environmental monitoring and protection: 4
5. Challenges and opportunities: 4
1. What is AI in Green Computing?
- AI-powered green computing involves the use of machine learning (ML), deep learning (DL), and AI algorithms to make computing systems more energy-efficient, environmentally friendly, and sustainable. AI helps optimize power usage, reduce waste, and improve resource management in various computing environments.
2. Major applications of AI in green computing
- Energy-efficient data centers
- Data centers consume massive amounts of electricity, and AI can improve energy usage:
- Dynamic cooling optimization: AI-powered cooling systems adjust in real-time to reduce energy waste (e.g., Google’s AI-powered cooling system reduced energy usage by 40%).
- Workload distribution: AI effectively balances the workload across all servers to reduce energy consumption.
- Predictive maintenance: AI detects hardware failures early, reducing energy downtime.
- Smart grids and renewable energy integration
- AI improves the smart grid by:
- Forecasting energy demand: AI models predict when electricity is needed to improve grid efficiency.
- Improving renewable energy use: Integrating solar and wind power into computing operations for AI sustainability.
- Reducing carbon footprint: AI enables carbon-conscious computing and schedules energy-related tasks when green energy is available.
- AI in hardware optimization
- Energy-efficient chip design: AI helps design chips that consume less power while maintaining performance (e.g., NVIDIA and ARM use AI for low-power chips).
- Self-learning processors: AI-powered processors dynamically adjust power consumption based on usage.
- D. AI for sustainable software development
- Code optimization: AI optimizes software code to perform tasks using minimal resources.
- Green AI models: AI models are being trained with reduced computational requirements (e.g., OpenAI’s performance-focused training methods).
- EA in e-waste management
- AI-powered recycling: AI improves sorting and recycling of e-waste.
- Predictive analytics for lifecycle management: AI predicts the lifespan of hardware to encourage reuse and premature disposal.
3. Benefits of AI in green computing
- Energy savings: AI reduces energy consumption in data centers, leading to lower electricity bills and carbon emissions.
- Lower carbon footprint: AI ensures that computing resources are used efficiently, reducing environmental impact.
- Cost reduction: Businesses save money on energy bills, maintenance, and hardware replacement.
- Increased hardware longevity: AI-powered predictive maintenance reduces hardware failures and increases its lifespan.
- Optimized cloud computing: AI ensures that cloud services operate efficiently with minimal energy wastage.
4. Challenges of AI in green computing
- High upfront costs: Implementing AI for green computing requires significant investment in infrastructure.
- Data privacy concerns: AI-driven optimization involves data collection, which raises privacy concerns.
- Energy consumption of AI: Training large AI models requires significant computing power, which raises environmental concerns.
- Lack of standardization: There is no global standard for AI-based green computing methods.
5. The future of AI in green computing
AI-powered carbon-neutral computing: Companies aim to achieve 100% carbon-neutral operations using AI.
- Quantum computing and AI: AI-powered quantum computing could revolutionize energy efficiency.
- AI-optimized edge computing: Reducing dependence on centralized cloud computing for lower energy consumption.
- Self-sustaining AI models: AI models that continuously improve to achieve energy efficiency.
Conclusion
AI in green computing is revolutionizing the way technology uses energy, manages resources, and reduces environmental impact. By optimizing energy usage in data centers, improving hardware performance, and reducing e-waste, AI is paving the way for a more sustainable digital future.
_________________________________________________
Here are 10 key takeaways from “AI in Green Computing”:
- Environmental impact of AI: The increasing use of AI leads to increased energy consumption and carbon emissions, especially in data centers.
- Green computing as a solution: Green computing aims to use computing resources efficiently while reducing environmental impact, making AI more environmentally friendly.
- Algorithm optimization: Fine-tuning AI models can reduce computational demands without sacrificing performance, meaning more efficient use of resources.
- Energy-efficient hardware: Developing specialized processors (such as GPUs) that prioritize low power consumption for AI tasks.
- Renewable energy for data centers: Powering data centers with solar, wind, or hydroelectric power to minimize the carbon footprint.
- Edge computing: Processing data closer to the source saves energy, reducing the need for large data centers.
- Innovations in data center cooling: Implementing advanced cooling systems to reduce energy consumption and maintain optimal temperatures.
- Lifecycle and e-waste reduction: Extending the lifespan of AI hardware and recycling responsibly to reduce e-waste.
- AI in sustainable practices: Using AI to improve resource allocation, reduce waste, and improve efficiency in sectors such as agriculture, energy, and transportation.
- Policies and future: Governments and organizations create policies to promote green computing practices in AI.
________________________________________________
White Pages Research on “AI in Green Computing”
Introduction:
Artificial intelligence (AI) and green computing are an interconnected field that has immense potential to address the growing environmental concerns associated with technology. This research explores various approaches to promoting sustainability in computing, from optimizing energy consumption to improving resource management.
Key Focus Areas:
1. Energy Efficiency in AI Systems:
-
- AI algorithms can be optimized to reduce computational demands and energy consumption.
- Specialized hardware, such as GPUs, can be designed for energy-efficient AI processing.
- AI can be used to manage and optimize energy consumption in data centers.
2. AI for Sustainable Data Centers:
-
- AI can monitor and control cooling systems in data centers, reducing energy waste.
- AI can predict and prevent equipment failures, minimizing loss of time and energy.
- AI can optimize server utilization, ensuring efficient use of resources.
3. AI in resource management:
-
- AI can be used to improve resource allocation in cloud computing environments.
- AI can improve waste management and recycling processes.
- AI can help develop sustainable supply chains.
4. AI for environmental monitoring and protection:
-
- AI can analyze environmental data to identify patterns and predict environmental changes.
- AI can be used for wildlife monitoring and conservation efforts.
- AI can help develop sustainable agricultural practices.
5. Challenges and opportunities:
-
- Development of energy-efficient AI algorithms and hardware.
- The need for standardization and best practices in green AI.
Ethical considerations of using AI for environmental purposes.
Assumption:
AI has the potential to be a powerful tool in the fight for environmental sustainability. By leveraging AI in green computing, we can create a future where technology and environmental responsibility go hand in hand.
Additional Research:
-
- Explore the latest advances in energy-efficient AI hardware and algorithms.
- Investigate the role of AI in promoting sustainable practices across various industries.
- Examine the ethical implications of using AI for environmental purposes.
Note: This research is based on currently available information and may evolve as the field of AI in green computing continues to mature.
________________________________________