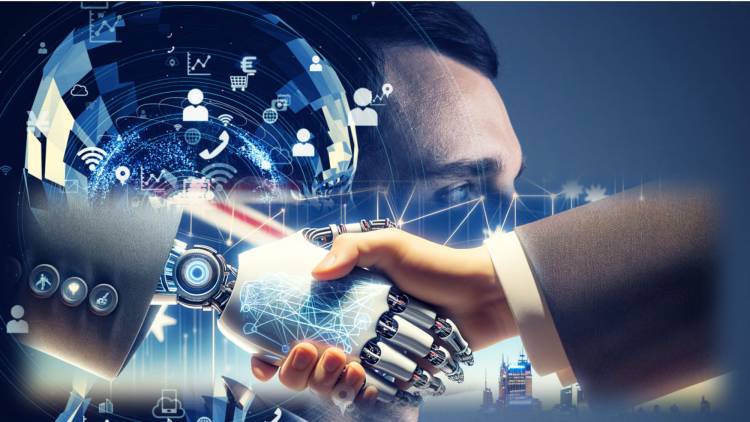
Increasing public trust in AI: A multifaceted challenge
Increasing public trust in AI: A multifaceted challenge
Building public trust in artificial intelligence (AI) is a complex problem with no easy solution. This requires a concerted effort from a variety of stakeholders, including developers, businesses, governments, and the public.
Here’s a breakdown of the key aspects:
Table of Contents
Increasing public trust in AI: A multifaceted challenge. 1
1. Understanding the trust deficit: 1
2. Key strategies for building trust: 1
3. Role of different stakeholders: 1
5. Challenges and opportunities: 2
Amazing Points on How to Increase Public Trust in AI 2
10 of the most important points about increasing public trust in AI: 3
Research work about Increased public trust with AI 4
1. Factors that affect trust: 4
4. Challenges and future directions: 5
1. Understanding the trust deficit:
Public trust in AI is often hampered by:
- Lack of understanding: Many people don’t understand how AI works, leading to fear and doubt.
- Ethical concerns: Concerns about job displacement, bias, privacy breaches, and lack of accountability.
- Negative portrayal: The media often focuses on the potential risks of AI, fueling anxiety.
- Past failures: AI systems have made mistakes, reinforcing skepticism.
2. Key strategies for building trust:
- Transparency and clarity: AI systems should be designed to be understandable. Explainable AI (XAI) focuses on making AI decisions transparent and interpretable.
- Ethical development and deployment: Adhere to ethical guidelines and principles throughout the AI lifecycle, from development to deployment.
- Robustness and trustworthiness: Ensure AI systems are reliable, accurate, and safe, reducing errors and bias.
- Responsibility and accountability: Establish clear lines of responsibility for AI initiatives and their outcomes.
- Data privacy and security: Ensure the protection and security of personal data used by AI systems.
- Education and public engagement: Educate the public about AI capabilities and limitations, address concerns, and promote open dialogue.
- Collaboration and partnerships: Foster collaboration between researchers, developers, policymakers, and the public to address AI-related challenges.
3. Role of different stakeholders:
- Developers: Focus on building ethical, transparent, and trustworthy AI systems.
- Businesses: Use AI responsibly, prioritising ethical considerations and data privacy.
- Governments: Develop regulations and policies that promote the development and use of responsible AI.
- Public: Engage in informed conversations about AI, ask questions and demand accountability.
4. Example steps:
- AI ethical principles and guidelines: Many organizations have developed ethical frameworks for AI.
- XAI research and development: Focus on making AI decisions more transparent.
- Data privacy regulations: GDPR and other regulations aim to protect personal data.
- Public awareness campaigns: Initiatives to educate the public about AI.
5. Challenges and opportunities:
- Building public trust in AI is an ongoing challenge. This requires ongoing effort, adaptation and cooperation. However, the potential benefits of AI are enormous and by addressing the trust deficit we can harness them while mitigating the risks.
- In conclusion, building public trust in AI is a complex but important task. By prioritizing transparency, ethics, trustworthiness, and public participation, we can pave the way for a future where AI benefits everyone.
Amazing Points on How to Increase Public Trust in AI
While public trust in AI is a composite and emerging issue, here are 10 points that may seem incredible but highlight the potential for positive change:
- AI as a trust builder: Imagine AI systems so transparent and explainable that they actually build trust in institutions by showcasing their work and decision-making processes.
- AI for ethical decision-making: AI can be used to analyze large amounts of data to identify and reduce biases in human decision-making, resulting in better outcomes and greater trust in the system.
- AI as a watchdog: AI can be used to monitor other AI systems for ethical violations and biases, acting as an independent auditor and ensuring accountability.
- Personalized education using AI: AI can be used to create personalized educational programs that address individual concerns and misconceptions about AI, fostering understanding and trust.
- AI for public engagement: AI-powered platforms can facilitate open and inclusive dialogue about AI, gather public input, and ensure AI development aligns with societal values.
- AI-powered transparency initiatives: AI can be used to analyze and visualize complex data, making it easier for the public to understand government policies and corporate practices, promoting transparency and trust.
- AI for collaborative governance: AI can be used to analyze public opinion and feedback, helping governments make more informed and responsible decisions, increasing trust in governance.
- AI for personalized healthcare: AI can be used to provide personalized healthcare recommendations and treatments, improve patient outcomes, and increase trust in medical professionals.
- AI for environmental protection: AI can be used to monitor and analyze environmental data, helping us understand and address climate change and other environmental challenges, increasing trust in environmental efforts.
- AI for social good: AI can be used to address social issues such as poverty, inequality and disease, demonstrating its potential to create positive impact and increase public trust.
Note:
These points may seem incredible today, but they highlight the potential of AI to be a force for good and a means of building trust in various aspects of society. By focusing on ethical development, transparency and public engagement, we can move towards a future where AI is seen as a trusted partner in solving some of the world’s most pressing challenges.
10 of the most important points about increasing public trust in AI:
- Transparency is paramount: People need to understand how AI systems work, especially when those systems make decisions that affect their lives. “Black box” AI erodes trust. Explainable AI (XAI) is important.
- Ethics must be built in: AI development and deployment must be guided by sound ethical principles. This includes removing bias, ensuring impartiality, and protecting confidentiality. Ethical considerations should not be an afterthought, but a core component of the entire AI lifecycle.
- Accountability is essential: When AI systems make mistakes, there must be clear lines of responsibility. Who is responsible for the consequences of AI-based decisions? Establishing a clear accountability framework is crucial to building trust.
- Data privacy is non-negotiable: AI systems often rely on large amounts of data, including personal information. Protecting data privacy is absolutely essential. Strong data protection measures and compliance with privacy regulations are critical to maintaining public trust.
- Bias mitigation is important: AI systems can inherit and amplify biases present in data, leading to unfair or discriminatory outcomes. Proactively identifying and mitigating these biases is essential to ensuring fairness and building trust.
- Robustness and reliability are important: AI systems must operate reliably and consistently. Errors and unpredictable behavior can damage trust. Rigorous testing and validation are needed to ensure robustness.
- Public education is key: Many people have misconceptions about AI. Public education initiatives are needed to explain AI’s capabilities and limitations, allay fears, and promote informed discourse.
- Collaboration is key: Building trust in AI requires collaboration between researchers, developers, policymakers, businesses, and the public. Open communication and partnerships are essential to addressing the complex challenges related to AI.
- Regulation may be necessary: In some cases, regulation may be necessary to ensure that AI systems are developed and used responsibly. Regulations can help set standards and protect people from harm.
- Trust is earned, not given: Building public trust in AI is an ongoing process that requires constant effort and a clear commitment to ethical principles, transparency, and accountability. Trust is not given automatically. It must be earned and maintained.
Research work about Increased public trust with AI
Research on increasing public trust in AI is a growing field that explores the factors that influence trust and how to build it. Below is a brief overview of the key areas:
1. Factors that affect trust:
- Transparency and clarity: Studies show that understanding how AI works is crucial to building trust. Explainable AI (XAI) is a key focus.
- Ethical concerns: Research investigates public perceptions of AI ethics, including bias, fairness, privacy, and accountability.
- Competence and trustworthiness: People need to see that AI systems are accurate, trustworthy, and work as expected.
- Human qualities: Some research explores how anthropomorphism (giving AI human qualities) affects trust.
- Social and contextual factors: Trust can vary depending on the specific application, user background, and social norms.
2. Building trust:
Developing XAI: Researchers are working on ways to make AI decisions more transparent and interpretable.
AI ethical frameworks: The study explores the development and implementation of ethical guidelines and principles for AI.
Public engagement: Research explores how to effectively communicate about AI and engage the public in conversations about its implications.
Strategies for building trust: Studies examine various strategies for building trust, such as demonstrating the benefits of AI, ensuring accountability, and addressing concerns.
3. Key areas of research:
Measuring trust: Developing reliable ways to measure public trust in AI is crucial for tracking progress and evaluating interventions.
Trust in specific AI applications: Research explores trust in AI in various domains, such as healthcare, finance, and transportation.
The role of the media: Studies investigate how media portrayals of AI affect public perception and trust.
4. Challenges and future directions:
The dynamic nature of trust: Trust is not static; it can change over time and with new experiences. This requires research.
- The complexity of AI: Explaining complex AI systems in an understandable way is a challenge.
- Cultural differences: Trust in AI can vary across cultures, requiring research that considers these differences.
In short, research to increase public trust in AI is multidisciplinary, drawing from computer science, psychology, sociology, and other fields. The goal is to understand the factors that shape trust and develop strategies to ensure that AI is used in a way that benefits society.