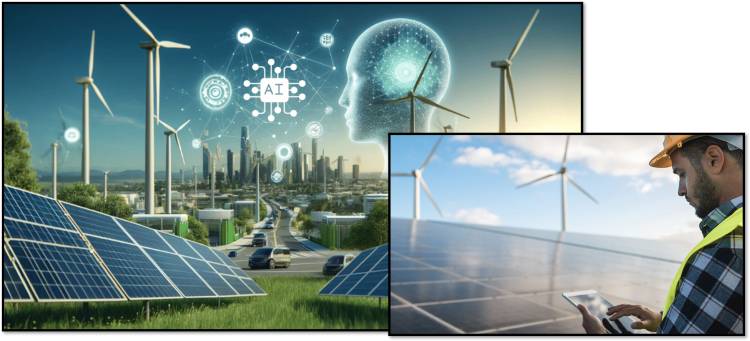
Renewable Energy Integration with AI
Renewable Energy Integration with AI: A Closer Look
Integrating renewable energy sources, such as solar and wind, into existing energy grids is crucial for a sustainable future. However, these sources are inherently intermittent, presenting significant challenges for grid stability and reliability. This is where artificial intelligence (AI) comes into play, offering powerful solutions to improve and streamline renewable energy integration.
Table of Contents
Renewable Energy Integration with AI: A Closer Look. 1
Key applications of AI in renewable energy integration: 2
1. Resource assessment and site selection: 2
3. Grid management and optimization: 2
Benefits of AI in renewable energy integration: 3
Here are 10 key takeaways on integrating renewable energy with AI: 3
2. Improves resource assessment: 3
3. Improves energy forecasting: 3
4. Improves grid management: 3
5. Enables predictive maintenance: 3
6. Facilitating smart grids: 3
7. Increased energy efficiency: 4
10. Data and expertise are needed. 4
White Paper on “Integrating Renewable Energy with AI”. 4
2. Applications of AI in renewable energy integration: 4
2.1 Resource assessment and site selection: 4
2.3 Grid management and optimization: 5
3. Benefits of AI-powered renewable energy integration: 5
4. Challenges and opportunities: 5
Key applications of AI in renewable energy integration:
1. Resource assessment and site selection:
- AI algorithms analyze a wide range of data, including satellite images, weather patterns, and topographical information, to identify the best locations for renewable energy installations, such as solar farms and wind farms.
- It ensures maximum energy production and a cost-effective investment.
2. Energy forecasting:
- AI models use machine learning to predict energy production with high accuracy by analyzing historical weather data, power generation records, and other relevant variables.
- Accurate forecasting is essential for grid operators to balance energy demand and supply, thereby ensuring grid stability.
3. Grid management and optimization:
- AI-powered systems monitor real-time data from multiple sources, including renewable generators and consumer demand, to optimize grid operations.
- AI algorithms make quick decisions to balance energy supply and demand, prevent grid overloads, and ensure consistent power supply.
4. Restoration forecasting:
- AI-powered systems monitor renewable energy assets in real-time, analyzing sensor data and performance metrics to identify potential failures or anomalies.
- Enables proactive maintenance, minimizes downtime, and maximizes equipment lifespan.
5. Smart grids:
- AI plays a key role in the development of smart grids, which are modern power grids that use digital technology to monitor and manage energy flows.
- AI algorithms optimize power distribution, improve grid resilience, and enable seamless integration of renewable energy sources.
Benefits of AI in renewable energy integration:
- Increased energy efficiency: AI optimizes renewable energy systems, ensuring maximum energy production and reducing waste.
- Improved grid stability: AI-powered grid management systems balance intermittent renewable energy production with demand, ensuring a continuous supply of electricity.
- Cost optimization: AI helps identify cost-effective locations for facilities and reduces maintenance costs through predictive analytics.
- Environmental sustainability: By promoting the use of renewable energy and optimizing energy consumption, AI contributes to a more sustainable energy landscape.
- Challenges and opportunities:
- Data availability and quality: Large amounts of high-quality data are required to effectively train AI algorithms.
- Model development and validation: Developing accurate and reliable AI models for renewable energy integration requires expertise and resources.
- Integration with existing infrastructure: Integrating AI-powered solutions with existing energy infrastructure can be complex.
Bottom line:
AI is revolutionizing the way we integrate renewable energy sources into our energy systems. By improving resource assessment, energy forecasting, grid management, and predictive maintenance, AI is making a more sustainable and reliable energy future possible. As AI technology advances, its role in renewable energy integration will only grow, paving the way for a cleaner, greener world.
Here are 10 key takeaways on integrating renewable energy with AI:
- Intermittent detection: AI helps manage fluctuations in renewable sources such as solar and wind, ensuring grid stability.
- Improves resource assessment: AI analyzes data to identify ideal locations for renewable energy installations.
- Improves energy forecasting: AI predicts renewable energy production more accurately, helping with grid management.
- Improves grid management: AI-powered systems balance energy supply and demand in real-time for efficient distribution.
- Enables predictive maintenance: AI identifies potential equipment failures, minimizing downtime and maximizing lifespan.
- Facilitating smart grids: AI is critical to developing smart grids that optimize energy flows and seamlessly integrate renewable sources.
- Increased energy efficiency: AI maximizes energy production from renewable sources and reduces waste.
- Reduces costs: AI helps optimize investments in renewable energy and reduces maintenance costs.
- Promotes sustainability: AI accelerates the adoption of clean energy sources, contributing to a greener future.
- Data and expertise are needed: Effective implementation of AI depends on high-quality data and specialized knowledge.
White Paper on “Integrating Renewable Energy with AI”
White Paper: Integrating Renewable Energy with AI
Abstract:
The growing reliance on renewable energy sources, such as solar and wind, is critical to a sustainable energy future. However, their intermittent nature presents significant challenges to grid stability and reliability. This white paper explores how artificial intelligence (AI) is revolutionizing renewable energy integration by providing innovative solutions for resource assessment, energy forecasting, grid management, predictive maintenance, and smart grid development. It examines the benefits, challenges, and future opportunities of this intersection, highlighting the potential for AI to accelerate the transition to a clean energy landscape.
1. Introduction:
The global shift to renewable energy is driven by the urgent need to mitigate climate change and ensure energy security. While renewables offer numerous environmental and economic benefits, their inherent intermittency (fluctuating based on weather patterns and time of day) requires sophisticated management strategies. AI, with its ability to process large data sets, identify complex patterns, and make decisions in real-time, has emerged as a key enabler for successful renewable energy integration.
2. Applications of AI in renewable energy integration:
2.1 Resource assessment and site selection:
- AI algorithms analyze various data sources to identify the best locations for renewable energy installations, including satellite images, weather data, topographical information, and historical records of energy production. This process maximizes energy production while minimizing environmental impact.
2.2 Energy forecasting:
- Accurate forecasting of renewable energy production is crucial for grid operators to balance supply and demand. AI models, specifically machine learning algorithms, combine historical weather data, real-time sensor readings, and other relevant variables to predict energy production with high accuracy. This improves grid stability and reduces reliance on backup energy sources.
2.3 Grid management and optimization:
- AI-powered systems monitor real-time data from various points on the grid, including renewable energy generators, conventional power plants, and consumer demand. AI algorithms improve grid operations by dynamically adjusting power flows, balancing supply and demand, and avoiding grid congestion or blackouts.
2.4 Predictive maintenance:
- AI plays a critical role in predictive maintenance by analyzing sensor data and performance metrics from renewable energy assets such as wind turbines and solar panels. By identifying anomalies and potential failures, AI enables proactive maintenance, reducing downtime, extending equipment life, and lowering operating costs.
2.5 Smart grids:
- AI is the cornerstone of smart grid development. Smart grids use digital technologies to monitor and manage energy flows in real time. AI algorithms improve power distribution, increase grid resilience to disruptions, facilitate the integration of distributed energy resources, and enable consumers to effectively manage their energy consumption.
3. Benefits of AI-powered renewable energy integration:
- Increased grid stability: AI mitigates the challenges posed by intermittent renewable generation, ensuring reliable power supply.
- Increased energy efficiency: AI improves energy production from renewable sources and reduces energy losses in transmission and distribution.
- Cost optimization: AI helps reduce capital costs through optimized site selection and reduces operating costs through predictive maintenance.
- Accelerate renewable energy adoption: By improving the reliability and cost-effectiveness of renewable energy, AI encourages broader adoption and accelerates the transition to a sustainable energy system.
- Environmental sustainability: AI promotes the use of clean energy sources, reduces greenhouse gas emissions, and mitigates climate change.
4. Challenges and opportunities:
- Data availability and quality: AI algorithms require large amounts of high-quality data for training. Ensuring data availability and accuracy is crucial for successful implementation.
Decision (Research Page):
AI is changing the landscape of renewable energy integration, offering powerful tools to address the challenges of intermittency and grid management. By improving resource assessment, enhancing energy forecasting, optimizing grid operations, and enabling predictive maintenance, AI is paving the way for a more sustainable and reliable energy future. Continued research and development in AI, along with supportive policies and investments, will be crucial to unlocking the full potential of AI and accelerating the transition to a clean energy economy.
____________________________________________________________________