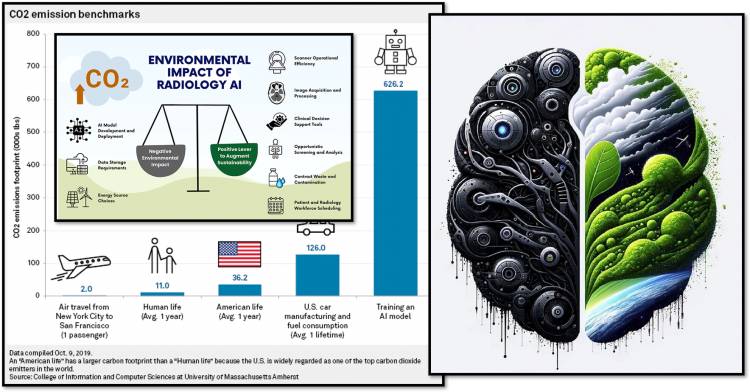
Reducing the environmental impact of detailed AI
Reducing the environmental impact of detailed AI insights
The ecological impact of AI is a complex topic with potential benefits and drawbacks.
Table of Contents
Reducing the environmental impact of detailed AI insights. 1
Reducing negative influences. 1
10 Key Points to Reduce the Environmental Impact of AI 2
“Reducing the Environmental Impact of AI” Research Page. 3
The Environmental Costs of AI 3
Strategies to reduce environmental impact 3
Links for More Information about Reduced environmental impact of AI 4
Opposite properties
- Energy consumption: Training and running AI models, especially large language models, requires huge amounts of energy, often obtained from fossil fuels. This contributes to greenhouse gas emissions and climate change.
- E-waste: Manufacturing and disposing of AI hardware generates e-waste, which contains harmful chemicals that can pollute the environment.
- Water habit: Cooling data centers that house AI systems require significant amounts of fresh water, which can deplete local resources.
- Resource scarcity: Manufacturing AI hardware requires rare earth minerals and other resources, which can lead to habitat destruction and environmental degradation.
Optimistic things
Climate modeling: AI can be used to develop more accurate climate models, which can help us better understand and predict the impacts of climate change.
- Renewable energy optimization: AI can be used to improve the action of renewable energy systems, such as solar and wind power plants, making them more efficient and cost-effective.
- Sustainable agriculture: AI can be used to develop more sustainable farming practices, such as precision agriculture, which can reduce the use of water, fertilizers, and pesticides.
- Conservation efforts: AI can be used to monitor and protect endangered species, detect deforestation, and combat illegal wildlife trade.
Reducing negative influences
- Improving energy efficiency: Researchers are working on developing more energy-efficient AI algorithms and hardware.
- Transitioning to renewable energy: Data centers can run on renewable energy bases, such as solar and wind, to reduce their carbon footprint.
- Recycling and reuse: We need to improve recycling and reuse of AI hardware to reduce e-waste.
- Developing sustainable AI hardware: Researchers are working on developing AI hardware that uses less energy and fewer resources.
Deduction
AI has the potential to be a powerful tool to address the world’s most pressing environmental challenges. However, it is important to be aware of the potential negative impacts of AI and take steps to mitigate them. By investing in research and development, promoting sustainable practices, and raising awareness, we can ensure that AI is used in a way that benefits both humanity and the environment.
10 Key Points to Reduce the Environmental Impact of AI
- Advance model act: Develop AI models that achieve similar accuracy with smaller, less complex designs. This reduces the computational capitals and energy required for training and operation.
- Use renewable energy: Power data centers and AI operations with renewable energy sources such as solar, wind, and hydroelectric to reduce dependence on fossil fuels and cut carbon emissions.
- Take advantage of cloud-based solutions: Use cloud platforms that offer economies of scale and improved data center design for energy efficiency. Cloud providers often invest in renewable energy and efficient cooling systems.
- Improve hardware performance: Advance hardware technology to create more energy-efficient processors and chips. This includes the search for new materials, innovative chip architectures, and quantum computing.
- Implement software optimization: Use techniques such as model pruning, quantization, and knowledge distillation to make AI models more efficient in terms of memory usage and processing power.
- Reduce data storage requirements: Develop strategies to minimize the amount of data needed for training and operations, as data storage also consumes energy.
- Minimize training frequency: Explore methods such as Retrieval-Augmented Generation (RAG) that allow models to learn from new information without the need for complete retraining, thereby saving energy.
- Plan for longevity: Create AI models that are adaptable and can be used for long periods of time without the need for frequent retraining or replacement, thereby reducing overall environmental impact.
- Promote responsible AI practices: Encourage the development and use of AI in a way that prioritizes environmental sustainability and minimizes negative impacts.
- Measure and monitor environmental impact: Develop standardized methods to assess the environmental impact of AI systems, track energy consumption, and identify areas for improvement.
“Reducing the Environmental Impact of AI” Research Page
Absolutely! Below is an outline of a research page focused on reducing the environmental impact of AI, including key points and potential research directions:
Reducing the Environmental Impact of AI: A Research Agenda
Introduction
Artificial intelligence (AI) has become a transformative force across industries, but its increasing prevalence raises concerns about its environmental impact. This research page explores the environmental costs associated with AI, examines strategies to mitigate these impacts, and proposes a research agenda for developing sustainable AI practices.
The Environmental Costs of AI
- Energy Consumption: Training and running AI, especially for large models, requires significant computational power, resulting in considerable energy consumption and greenhouse gas emissions.
- Hardware Production and E-Waste: Production of AI-related hardware (processors, servers, etc.) involves resource extraction, energy use, and the production of e-waste.
- Water usage: Data centers, which house AI infrastructure, use large amounts of water for cooling purposes.
- Lifecycle impacts: A comprehensive taxation of the environmental impacts of AI must consider the entire lifecycle, from resource extraction to end-of-life disposal.
Strategies to reduce environmental impact
- Improve model performance
- Research to develop smaller, more efficient AI models that achieve comparable performance with fewer computational resources.
- Explore techniques such as model pruning, quantification, and knowledge extraction to minimize energy consumption during training and estimation.
- Use of renewable energy
- Investigate the feasibility and scalability of powering AI operations with renewable energy sources (solar, wind, hydroelectricity).
- Analyze the role of cloud computing providers in promoting sustainable AI practices through their investments in renewable energy infrastructure.
- Improving hardware performance
- Supporting research and development of energy-efficient processors, chips, and hardware architectures tailored for AI workloads.
- Exploring the potential of emerging technologies such as quantum computing and neuromorphic computing to reduce energy consumption.
- Software optimization
- Developing software tools and libraries that facilitate the development and deployment of energy-efficient AI.
- Researching techniques to optimize AI algorithms and code to minimize resource usage.
- Data management and storage
- Investigating ways to reduce data storage requirements through techniques such as data compression, deduplication, and efficient data selection.
- Exploring the environmental impact of data storage and transfer and strategies to minimize it.
- Responsible AI behavior
- Developing ethical guidelines and best practices for the development and deployment of sustainable AI.
- Promote awareness and education about the environmental impacts of AI among researchers, developers, and users.
Research Guidelines
- Metrics and Measurement: Develop standard metrics and methodologies to accurately measure the environmental impact of AI systems.
- Lifecycle Assessment: Conduct comprehensive lifecycle assessments of AI technologies to identify hotspots and areas for improvement.
- AI for Sustainability: Investigate the potential of AI to contribute to environmental sustainability in areas such as climate modeling, resource management, and energy efficiency.
- Policy and Regulation: Explore the role of policy and regulation in promoting sustainable AI practices and fostering the development of environmentally responsible AI.
Conclusion
Reducing the ecological influence of AI is crucial to ensuring its long-term sustainability. By prioritizing research in the areas drawn above, we can pave the way for the growth of AI technologies that are both powerful and environmentally responsible.
Links for More Information about Reduced environmental impact of AI
https://libguides.ecu.edu/c.php?g=1395131&p=10318505
https://www.bakertilly.com/insights/the-environmental-impacts-of-ai
https://www.weforum.org/stories/2024/07/generative-ai-energy-emissions/
________________________________________________