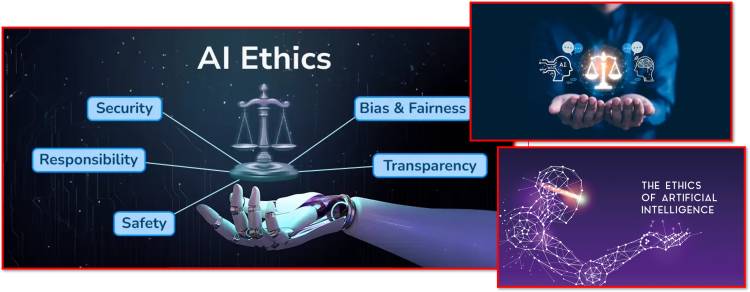
The Ethics of Artificial Intelligence: Issues and Measures
"The Ethics of Artificial Intelligence: Issues and Initiatives" Detailed information
The Ethics of Artificial Intelligence: Issues and Measures
Artificial intelligence (AI) is transforming industries, societies, and lives. However, the rapid adoption of AI raises significant ethical concerns. Addressing these challenges requires a balanced approach to innovation, justice, responsibility, and social welfare.
________________________________________________
Table of Contents
"The Ethics of Artificial Intelligence: Issues and Initiatives" Detailed information. 1
The Ethics of Artificial Intelligence: Issues and Measures. 1
Key ethical issues in artificial intelligence. 1
2. Privacy and surveillance: 1
3. Accountability and transparency: 1
4. Job displacement and economic impacts: 1
6. Disinformation and manipulation: 2
1. Global ethical guidelines: 2
4. Corporate responsibility: 2
5. AI research organizations: 2
6. Public awareness and education: 2
Future directions in AI ethics. 3
1. Regulation and legislation: 3
2. Multi-stakeholder collaboration: 3
Key components of sustainable AI 3
1. Environmental sustainability. 3
More Challenges to achieving sustainable AI 4
Strategies for sustainable AI 4
2. Renewable energy integration: 4
5. Circular economy for AI hardware: 5
6. Transparency and reporting: 5
1. Reduced environmental impact: 5
3. Google Carbon Neutral Data Centers: 6
4. UN AI for Good Initiative: 6
The Future of Sustainable AI 6
Key ethical issues in artificial intelligence
1. Bias and fairness:
- AI systems can acquire biases from the data they are trained on, leading to discrimination based on race, gender, age, or other factors.
- Example: Facial recognition algorithms misidentify certain demographics more frequently.
More... Bias and fairness with AI
2. Privacy and surveillance:
- AI's ability to analyze large amounts of data raises privacy concerns.
- Example: AI-based surveillance systems monitoring people without consent.
More... Privacy and surveillance in the age of AI
3. Accountability and transparency:
- The “black box” nature of many AI algorithms makes their decisions difficult to understand or explain.
- Who is responsible when AI makes a harmful decision: developers, users, or AI itself?
More ...Accountability and transparency with detailed AI visions
4. Job displacement and economic impacts:
- AI-driven automation threatens traditional jobs, especially in manufacturing and services.
- Ethical concerns arise regarding economic inequality and the need to retrain workers.
More: Job displacement and the economic impacts of AI
5. AI as a weapon:
- The development of AI-powered weapons and autonomous systems poses risks of misuse and escalation of conflict.
- Ethical questions surround machines that delegate life-or-death decisions.
More info:Weaponizing AI: Advantage: Disadvantages
6. Disinformation and manipulation:
- AI-generated content (e.g., deepfakes) can spread misinformation, fracture public opinion, and erode trust.
- Example: Fake videos that influence elections or social discourse.
More: Disinformation and AI manipulation
7. Human-AI interaction:
- Over-reliance on AI can reduce critical thinking and human autonomy.
- Ethical concerns include how AI systems are integrated into decision-making processes.
________________________________________________
Steps to address AI ethics
1. Global ethical guidelines:
- The UNESCO Recommendation on the Ethics of Artificial Intelligence sets international standards for the development and use of AI.
- Focus areas include human rights, environmental sustainability, and cultural diversity.
More: global ethical guidelines for AI
2. AI for good:
- The AI for Good Global Summit promotes AI applications that solve global challenges, such as poverty, education, and healthcare.
More: AI for Good important information
3. AI governance framework:
- The European Union has established guidelines for trustworthy AI, emphasizing accountability, transparency, and fairness.
- Countries such as Singapore and Canada have launched frameworks to drive AI implementation in a responsible manner.
More: AI Governance Framework:
4. Corporate responsibility:
- Companies such as Google, Microsoft, and IBM have published AI ethics principles focused on bias reduction, transparency, and responsible innovation.
More: Corporate Responsibility for AI
5. AI research organizations:
- Organizations such as Partnership on AI and OpenAI advocate for ethical research and promote awareness of the implications of AI.
More: AI Research Organizations
6. Public awareness and education:
- Measures to educate the public about the potential risks and benefits of AI.
- Programs aim to equip people with the knowledge needed to navigate a world driven by artificial intelligence.
More: Public awareness and education about AI
________________________________________________________________
Future directions in AI ethics
1. Regulation and legislation:
- Governments need to create strong laws to address the ethical challenges of AI without stifling innovation.
More:Regulation and legislation in AI Moral
2. Multi-stakeholder collaboration:
- Collaboration between governments, businesses, academia, and civil society is essential for effective ethical governance of AI.
More: Multi-Stakeholder Collaboration on AI info
3. AI literacy:
- Promoting AI literacy ensures that people and societies understand the technology and its implications.
More: AI Literacy: extensive information
4. Sustainable AI:
- Reducing the carbon footprint of AI and ensuring that its deployment is aligned with environmental goals.
More: Sustainable AI: Detailed info
More info of Sustainable AI: Building environmentally responsible AI
Sustainable AI refers to the development, deployment, and use of AI systems in ways that minimize environmental impacts while ensuring equitable social and economic outcomes. As AI continues to grow in importance, concerns arise about its energy consumption, resource usage, and broader environmental impact. Sustainable AI emphasizes creating systems that are not only efficient and effective, but also environmentally conscious and socially responsible.
Key components of sustainable AI
1. Environmental sustainability
- Energy efficiency: AI models, especially large-scale models like GPT-4 or DALL-E, consume significant computational resources, leading to high energy consumption. Designing more energy-efficient algorithms and using green data centers can reduce this impact.
- Carbon footprint reduction: Training and running AI models can emit tons of CO₂. The transition to renewable energy sources is crucial to powering AI infrastructure.
- Lifecycle assessment: Considering environmental impacts at every stage of AI development, from hardware manufacturing to model disposal.
More: Environmental Sustainability with AI
2. Social sustainability
- Equality and access: Ensuring AI systems are designed to benefit everyone, avoiding exclusivity due to technical or cost barriers.
- Fair labor practices: Ethical sourcing of materials for hardware and fair treatment of workers involved in the AI development and manufacturing process.
More: Social Sustainability with AI
3. Economic sustainability
- Cost-effective innovation: Balancing technological advances with cost-effective solutions that do not exploit limited resources.
- Job creation and reskilling: Addressing job displacement caused by AI automation through reskilling programs and promoting new industries.
More: Economic Sustainability with AI
More Challenges to achieving sustainable AI
1. High energy consumption:
- Training large AI models requires enormous computational power and consumes energy equivalent to the annual consumption of hundreds of homes.
- Example: GPT-3 training is reported to emit around 500 metric tons of CO₂.
More: High energy consumption with AI
2. E-waste from hardware:
- AI systems rely on high-performance computing hardware, leading to increased waste of electronic devices when components are discarded or replaced.
More: AI Hardware E-Waste
3. Unequal access:
- Developing countries may lack the resources needed to implement sustainable AI practices, which will widen the global technology gap.
More: Unequal access with AI
4. Balance of trade:
- Optimizing for sustainability may conflict with performance goals or development timelines.
More: AI Trade Balance
Strategies for sustainable AI
1. Green computing:
- Use energy-efficient chips and hardware.
- Improve AI algorithms to reduce computational demands.
More: AI in Green Computing
2. Renewable energy integration:
- Power data centers with solar, wind, or other renewable energy sources.
More:Renewable Energy Integration with AI
3. Model optimization:
- Use techniques such as model distillation or pruning to reduce the size of AI models while maintaining performance.
More: AI Model Optimization
4. Federated learning:
- Decentralize AI training to reduce the need for large centralized data centers and associated energy costs.
5. Circular economy for AI hardware:
- Recycle and reuse old components to minimize waste and raw material use.
More:Circular economy for AI hardware
6. Transparency and reporting:
- Organizations should publish the environmental impact of their AI projects and commit to sustainability goals.
More: AI Transparency and Reporting
Benefits of sustainable AI
1. Reduced environmental impact:
- Reduced carbon emissions and minimal resource depletion.
More:Reducing the environmental impact of detailed AI
2. Cost savings:
- Energy-efficient systems reduce operating costs over time.
More: AI’s Cost Savings:
3. Increased public trust:
- Demonstrating a commitment to sustainability can improve public perception and acceptance of AI technologies.
More: Increasing public trust in AI: A multifaceted challenge
4. Global equality:
- Making AI more accessible and sustainable ensures a fair distribution of its benefits across all nations.
More: The global AI equity
Real-world initiatives in sustainable AI
1. Green AI movement:
- Researchers advocate prioritizing energy-efficient AI models rather than simply maximizing performance.
2. Sustainability goals
Microsoft:
- Committed to being carbon negative by 2030 and introduced AI solutions for environmental monitoring.
3. Google Carbon Neutral Data Centers:
- Transitioning to 100% renewable energy and developing more energy-efficient machine learning tools.
4. UN AI for Good Initiative:
- Encourages the use of AI to address environmental challenges, such as climate change and biodiversity loss.
The Future of Sustainable AI
- Policy Development: Governments and international organizations need to implement regulations that promote sustainable AI practices.
- Hardware Innovation: Developing low-power computing devices to support AI while reducing energy consumption.
- Collaborative Efforts: Promoting and implementing multi-stakeholder collaboration between academia, industry, and environmental organizations.
________________________________________________
Related Topics:
Bias and fairness with AI
Privacy and surveillance in the age of AI
Accountability and transparency with detailed AI visions
Job displacement and the economic impacts of AI
Weaponizing AI: Advantage: Disadvantages
Disinformation and AI manipulation
global ethical guidelines for AI
AI for Good important information
Corporate Responsibility for AI
Public awareness and education about AI
Regulation and legislation in AI Moral
Multi-Stakeholder Collaboration on AI info
AI Literacy: extensive information
Environmental Sustainability with AI
Economic Sustainability with AI
High energy consumption with AI
Renewable Energy Integration with AI
Circular economy for AI hardware
Reducing the environmental impact of detailed AI
Increasing public trust in AI: A multifaceted challenge
_________________________________________________________________